Unlock Fear
Unlock the Secret of Acrophobia
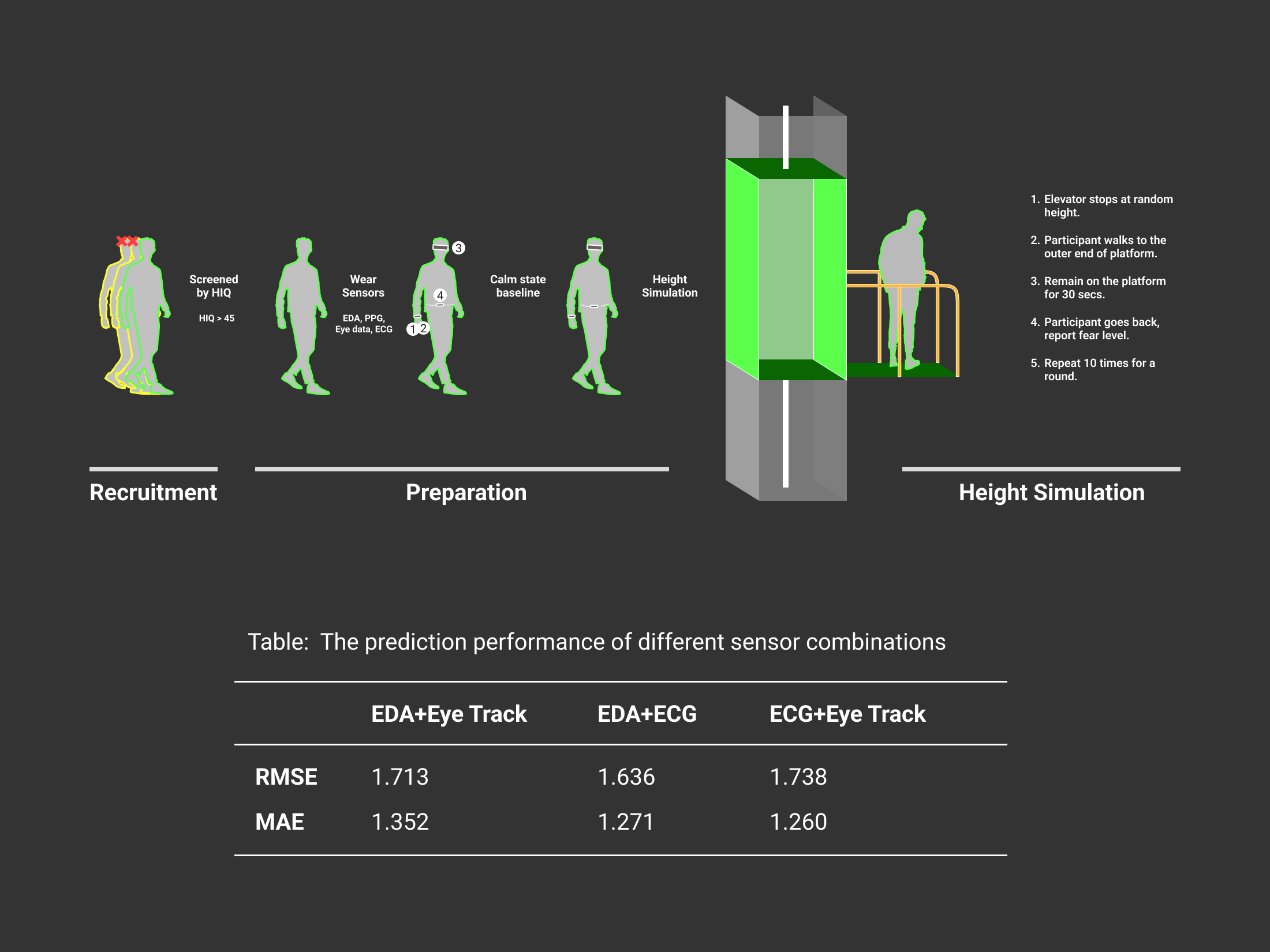


Overview
“Unlock the secret of fear”
Acrophobia, the fear of heights, significantly affects daily activities, career choices, and overall quality of life. People who suffer from acrophobia know this fear is excessive and unreasonable, yet 6.4% of total population suffer from this issue, according to DSM-5.
We investigate the feasibility of passive sensing data for fear measurement and discern the most influential physiological and behavioral indicators. This technology may fill the current gap for VR therapy, which shows promise in exposure therapy but requires ongoing monitoring and adjustments.
Role
Co-first author (of HCI research paper)
Duration
2022 July – 2023 Sept. (1 year 2 months)
Director
Yuntao Wang, Tsinghua PI HCI Lab
Category
Affective Computing, VR, Virtual Therapy
Status
Currently under review, IMWUT 24′
Acrophobia, or fear of heights, is a prevalent mental health condition that can profoundly impact daily activities, career choices, and overall quality of life. While virtual reality (VR) offers promising exposure therapy interventions, many rely on self-reported fear levels, demanding consistent monitoring and adjustments. This paper explores the potential of physiological and behavioral data to gauge real-time fear levels among acrophobia sufferers. We investigate the feasibility of passive sensing data for fear measurement and discern the most influential physiological and behavioral indicators. Through a VR study involving 25 participants, we collected physiological signals, gaze patterns, and subjective fear ratings. Our methodology attained an RMSE of 1.91 and MAE of 1.7 on a 10-point fear intensity scale, and we pinpointed significant factors affecting acrophobia severity measurements. Ultimately, our findings offer insights into more tailored and effective therapeutic strategies for acrophobia, enhancing the quality of life and holistic well-being of affected individuals.
Present methods show a good performance in the classification prediction task of acrophobia in a coarse-grained manner (2-4 classes). This implies the potential to further develop a fine-grained fear prediction model, which is desired by therapists and wasn’t mentioned in current studies.
Meanwhile, current prediction models mainly focus on internal physiological signals (EEG, EDA, HRV, etc.), which may be supplented and improved by adding in eye data, including pupil dilation and eye openess data.
Figure.1 shows the process of the data collection. During the data collection, we utilised two types of hardware devices: BIOPAC set and Vive Pro Eye. For BIOPAC set, we installed one BIOPAC station and attached two terminals to the participants (1 on the wrist, 1 on the chest). The terminal on the chest was used for collecting ECG data, while the other was used for recording EDA and PPG signals. Additionally, participants were asked to wear the Vive Pro Eye headset. Throughout the data collection, participants were instructed to wear VR headsets and keep the BIOPAC terminal attached to their bodies.
Preparation Before data collection, we synced wristbands and VR sets using a laptop. Participants donned BIOPAC devices, starting with a one-minute calm baseline. We recorded daily fear of heights and performance feedback. Participants experienced a realistic spatial perception in virtual reality with an elevator cabin and outside platform simulation.
Height Simulation Participants, after VR setup and eye calibration, experienced a height simulation. In a virtual elevator, they moved to random heights, exiting onto a platform and rating fear on a 1-10 scale. Elevator doors closed for 15 seconds, transitioning to another height (0m to 100m). Chosen to balance data, these heights align with heightened physiological and emotional responses near ground level and saturation around 100 meters. The experimenter observed fear-related behaviors, like trembling or altered stride length, during height exposure outside the elevator. This comprehensive approach aimed to capture nuanced reactions to simulated heights.
Session Repetition After returning to the elevator, it moved for 15 seconds before stopping at a new height. Participants repeated the platform-walking and fear-rating procedure. Three sessions, each on a different virtual site, comprised 10 heights each. Rests between sessions were allowed.
Data Overview Post-data collection, participants removed the device, and VR position recalibration occurred. Each participant produced 30 sets of data, encompassing 30-second records of 4 signal channels and behavioral traits. Entries, paired with self-reported fear scores (1-10), totaled 450 instances, forming a comprehensive dataset for analysis.
We recruited 46 participants from a local university using diverse social media advertisements. They completed the Heights Interpretation Questionnaire (HIQ) to assess height-related distress and avoidance. Those with HIQ scores ≤45 were excluded. Eleven participants dropped out, resulting in a final sample of 13 (5 females, 8 males, average age = 22.1, STD = 2.25).
Physiological and Activity Data In the experiment, participants wore the BioNomadix Wireless Transmitter and Vive Pro Eye. The BioNomadix has electrodermal activity (EDA), photoplethysmography (PPG), and Electrocardiograph (ECG) sensors. EDA captures skin conductivity changes, PPG measures blood volume pulse, and Vive Pro Eye records pupil size and eye openness at 90Hz.
Ground Truth Self-report Fear Level Data : This study employed self-report evaluations to gauge participants’ subjective fear responses to simulated high-altitude environments. Participants rated their fear level on a scale of 1 to 10 after each exposure, with 10 indicating the highest level of acrophobia.
Feature Extraction Features are derived from original data, using existing signal processing algorithm.
Traditional fear of height prediction employs classification or clustering, but in Virtual Reality Exposure Therapy (VRET), accurately measuring fear intensity is crucial. A regression-based approach, predicting continuous values, offers precise estimates, enabling personalized and timely feedback. This fine-grained fear prediction enhances VRET effectiveness in addressing acrophobia.
Ground Truth Self-reported fear of height scores (1-10) were adopted as ground truth, using a Verbal Rating Scale (VRS) for recording. VRS, deemed more suitable for its time efficiency and intuitiveness, was preferred over the previously used SAM in our task.
Regressors Our research develops regression models for precise fear prediction in height-related scenarios. Considering data from three modalities with numerous features, including correlated and potentially insignificant ones, demands a robust model for high-dimensional, non-linear situations. We employ three well-established regression models: Random Forest, Support Vector Regression (SVR), and LightGBM Regression. These models, especially ensemble ones like RF and LightGBM, enhance accuracy and reliability, crucial for predicting fear levels associated with heights.
Validation To optimize our models, we employ cross-validation for both cross-user and individual-based models. Using leave-one-out cross-validation on our small dataset (390 points, 30 per individual), we average metrics (MAE, RMSE) over subjects. Grid search in the sklearn library refines cross-user model parameters, influencing individual models.
Baseline and Metrics To assess our model, we compare it to three baseline methods. Mean averages fear scores within the train set, Random generates random scores, and Linear builds a basic regression model. All baselines undergo the same validation as prediction models for unbiased comparison. Metrics used include root mean squared error (RMSE) and mean absolute error (MAE), standard in regression tasks.
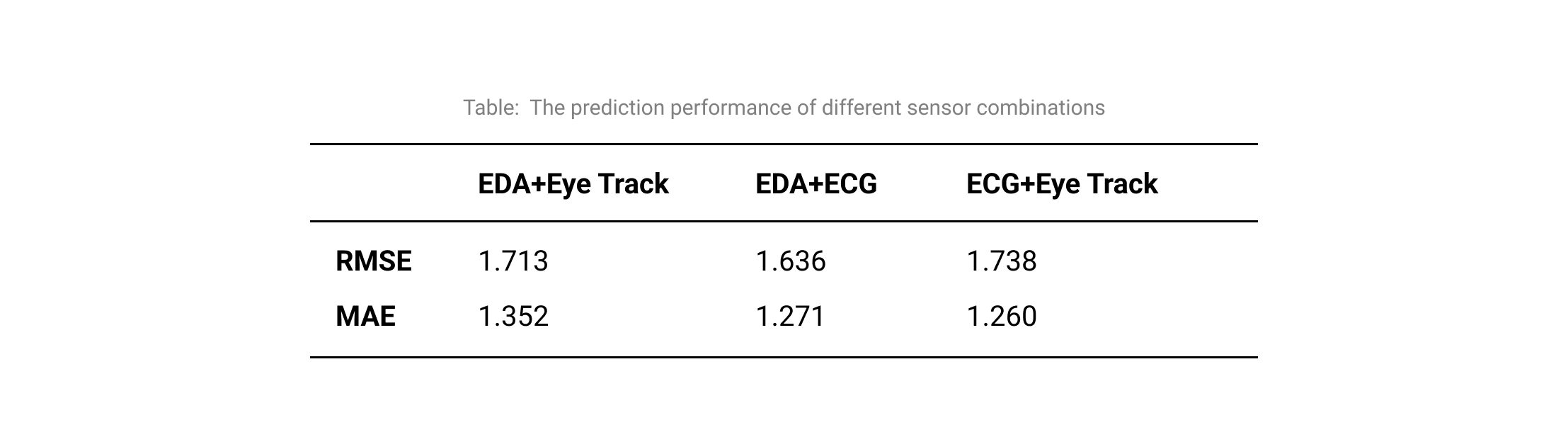